Last Updated: January 28, 2021
Every company does some type of forecasting, and although methodologies differ, the goal is the same: obtain the most accurate forecast possible to leverage and utilize resources most efficiently. Forecasting is important, and a lot is riding on it. A quality and insightful forecast can help a company make informed decisions that positively impact the business. A less than stellar forecast can lead to a series of bad decisions and cause irreparable damage. Artificial intelligence (AI) has proven to be an invaluable tool that can help companies improve forecast accuracy.
Understanding AI
AI or Machine Learning (ML – a subset of AI), is a segment of computer science that mimics cognitive processes in order to process data, or rather to learn or solve problems. Forecasting is a natural fit for ML. Through the analysis of information from various sources, ML can generate a thorough and complete forecast. Data points can include consumer data, such as website analytics, more traditional enterprise information via a business’s finance and operations departments, data from retailers, wholesalers, vendors or other partners and even information garnered from the Internet of Things (IoT) applications and sensors.
What’s At Stake
An incorrect or inaccurate forecast can have major consequences. Failing to properly identify competition, new retail channels, or a decrease in new stores for distribution can hurt sales and leave you with more inventory and less income than you expected. This, in turn, can impact reorder points and cash flow going forward. Increased costs, out-of-stock, and markdowns from overstocking are also very real possibilities resulting from a poor forecast that can negatively impact your bottom line and the health of your business.
Common Pitfalls
Forecasting is absolutely necessary to maintain a healthy business and to expand a business while also protecting a business from the volatility of the market. While forecasting is common, it is also easy to mess up. There are four common pitfalls companies tend to fall into when building their forecasting models.
Data Overload
Information is pivotal to forecasting, but too many data points can be overwhelming. Billions have been and are currently being invested in data initiatives by CPG and retail companies that have unleashed an ocean of data. When an employee is drowning in this ocean of data, it can be hard to separate or distinguish the true value of various facts and figures. Overwhelmed decision makers often fall back on gut-instinct or historical performance to guide category planning. While an overwhelmed team member may have valid reasons for making the decisions they do at that moment, their reactive response and gut-instinct is no match for data-driven findings.
Reacting to Competitor Moves
Retailers often gain market data (for example, point of sale, syndicated, or 3rd party data) to see trends in their industry and make forward-looking decisions. There is value to gauging the health of and direction an industry is taking, but the problem is that competitors are also making similar bets with limited information (often from the same sources and experts). While it is foolish to ignore your competitors, it is also important to not prioritize data gleaned from your competitors too significantly. Without understanding how your competitors are collecting and interpreting data, as well as what forecasting methodologies they are using, it is hard to understand this data completely.
Blindness to External Influences
Many organizations have either built or are building a robust Business Intelligence practice. However, traditional BI tools are great at accessing internal and historical data, such as past sales/revenue or marketing spend. They are not built to easily gather and analyze external data, such as economic changes or consumer behavior trends. Overwhelming resources would be needed to gain access to external data sources, like weather, consumer sentiment, unemployment figures, and so on. Building a repeatable process to acquire and leverage these sources on an ongoing basis requires dedicated time and effort.
Not Using Leading Indicators
Economists frequently utilize leading indicators to predict changes in an industry. These are early signs of interruption and indicate a shift in a particular direction. Organizations can use this same methodology to understand the headwinds and tailwinds of demand for a specific category. Too often, financial leaders rely simply on historical data to dictate future performance when it comes to demand, seasonality, etc. Without incorporating leading external indicators, a forecast is missing key influencers and has the potential to miss the mark completely. This has the potential to place a business behind their competition and fighting to regain ground and catch up.
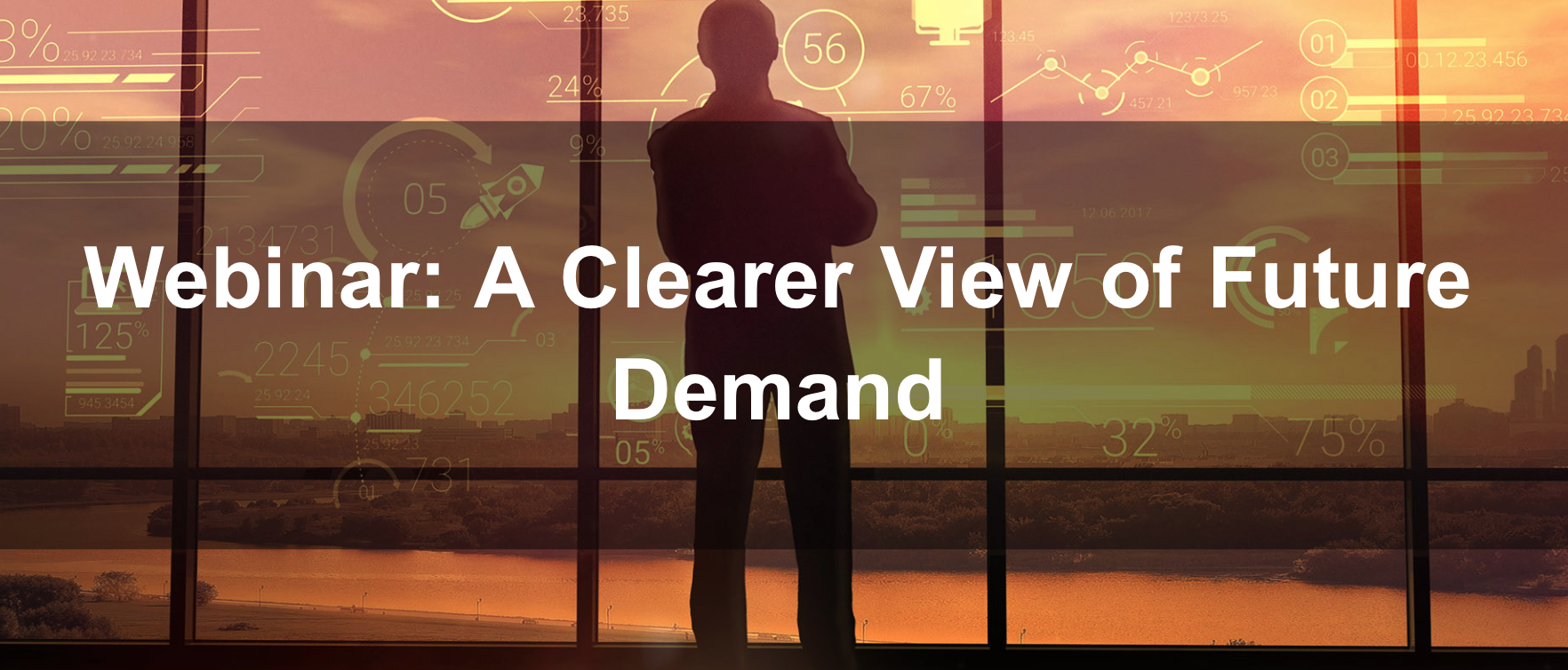
The Role of Machine Learning
ML enables companies to test multiple scenarios and validate hypothesis using a combination of internal data sources, like sales, shipments, and marketing spend; along with external data elements. When all of this information is combined, ML models have the potential to discern patterns and make accurate predictions about everything from shifting consumer opinions and habits, changes within the industry landscape, affects upcoming weather patterns will have on demand, and more.
All-Encompassing Platform
Pulling and processing different data points from various sources is a hassle and increases the odds of data fatigue or mishandling of information. ML makes it possible to track all data in one location. An example of this in practice is the Machine Learning technology being created and implemented by Prevedere (https://prevedere.com/). These all-inclusive platforms create a single location for data, eliminating miscommunication or compatibility issues between different platforms. Utilizing one platform typically requires easier maintenance and is also a more cost-effective solution.
The Significance of Timing
Forecasts are only useful if they are available in a timely manner. Businesses need time to interpret and react to a forecast. However, competently sorting through intensive, detail-rich data takes time. ML can quickly sort through decades worth of data, providing a concise analysis. As technology improves, the turnaround time between data acquisition and insight can be reduced even further.
Harnessing all of this data enables businesses to react in real time. A company can be agile by identifying trends that may have otherwise gone unnoticed, as well as hazards to avoid. The information provided by ML allows for accelerated responsiveness and reaction times, which improves efficiency and service throughout all departments, while also reducing costs.
Ability to Learn
Over time, organizations can begin to see the real power of ML as it repeatedly supplies additional information (i.e., data). With more data to train against, ML models improve accuracy by leveraging the existing and new datasets, identifying movements/tendencies and continue learning throughout the entire process.
Its learning capabilities enable ML models to demonstrate an understanding of the unique needs and requirements facing an industry and a specific business. This learned knowledge continues to be developed to help improve future forecasts. With refinement, these forecasts can become very tuned in to a specific business, enabling the technology to expertly leverage the available data to address the unique needs of that company.
Handling Anomalies
Anomalies are those little variances that have the potential to ruin an otherwise excellent forecast. Sometimes weird or unexplained things happen, but the thing with anomalies is that they are not always identifiable as such. Distinguishing between a freak anomaly and a bleeding-edge trend can be a challenge. Or rather, it can be a challenge when using traditional forecasting methods. ML forecasting systems can learn to spot anomalies for what they are and handle them with ease. Accurately defining an anomaly as such maintains the integrity and value of the forecast.
AI/ML Deterrents
Despite the clear benefits, the path to implementing an AI or ML solution is often difficult. Organization roadblocks, disagreement over taxonomy or rather the framework, along with lack of support from senior management and even uneven team support or understanding of the benefits all contribute to the difficulties of creating internal ML forecasting solutions. Even when everyone at a company is onboard with implementing ML forecasting solutions, finding the time to do so may be a roadblock. Many executives spend so much of their time handling routine tasks and responsibilities that finding time for strategic planning, no matter how much value this planning may deliver, can be a challenge. A recent survey conducted by Quest Mindshare in March of 2019, over half of the executives surveyed stated that they “lacked time to devote to strategic planning”.
Long-Term Solutions
Studies show many businesses currently direct some resources towards predictive analytics or AI at this time with more businesses planning to increase spending in this area in the future. Companies, however, are faced with the conundrum of deciding whether to build these solutions internally or outsource their AI needs. While an internal team may have the advantage of understanding the unique needs of their employer, working with a business that specializes in AI/ML technology and specifically forecasting may offer a better solution. An external partner may have a deeper understanding of the technology that enables a business to quickly implement effective solutions and often provide a quicker route to return on investment (ROI). If chosen correctly, the partner will work with internal subject matter experts (SMEs) to customize their technologies and systems to fit the specific needs of the business (i.e., forecasting shipments, revenue, demand).
Predicting Your Future
AI is the future of forecasting, and as the technology improves the forecasting capabilities of AI will continue to improve, as well. The right insights can inform business leaders to make important decisions. Capitalizing on AI can give CPG businesses an edge over their competition through properly leveraging and maximizing resources while also separating long-term trends from passing fads and providing even more valuable insights.
Investing in AI/ML empowers businesses to turn data into insights. By combining internal data with relevant, correlated external data sources, AI model forecasting can help you plan for tomorrow and transform your business for the better.
About Frank Mendoza
Frank Mendoza is CEO of Catalytics, a consulting firm that enables Fortune 500 companies to introduce emerging technologies, like AI and Advanced Analytics, to help create a more data-driven culture. Before founding Catalytics, he was an Executive Leader of the Services and Support Center of Excellence at 3M. Prior to working with 3M, Frank spent over a decade with Dell, serving in multiple roles in Strategy & Business Transformation, M&A, Manufacturing and Sales Operations. Read the original post: AI can Help CPG Companies improve Forecast Accuracy.