Last Updated: January 16, 2019
When it comes to big data and business intelligence, many companies are not making full use of their potential demand planning strategy.
Some companies are understaffed, lacking enough qualified data scientists or analysts to categorize and make sense of their data. Other organizations suffer from data processes that are not integrated, or too ad-hoc, keeping work siloed away and missing the big picture. Business intelligence teams may also find they lack the technology or resources to identify key insights.
Thankfully, with the new year comes a chance to reflect on what worked in the past and to set new professional or organizational goals. Below are three resolutions to consider for big data and business intelligence success in 2019.
3 Big Data and Business Intelligence Resolutions
1. Focus on tasks that add long-term value
A recent PwC study found that just 20% of a data scientist’s time is actually spent on work that is predictive. Often, data teams can get bogged down on the manual tasks of gathering, preparing and cleaning data that must be done before data can even be used effectively. While big data subscriptions like Prevedere’s Prevedere Indicators can greatly speed up the process of collecting and cleaning external data, ad-hoc requests can also distract from time spent on strategic tasks.
To focus on tasks that add long-term value, data teams need to ask if what they are doing aligns with their company’s overarching business goals. If the answer is yes, next they need to ask how their work will be used to drive decisions.
If tasks are not aligned with business goals, they should revisit the purpose behind those tasks by conferencing with business leaders on the outcomes they hope to achieve. That may kick off a conversation about direction and resources that data teams need to focus more on delivering strategic insights.
2. Account for external forces when forecasting
Internal data can reveal core insights about past practices or business intelligence decisions but over-relying on such data can leave critical gaps about external forces in the broader economy.
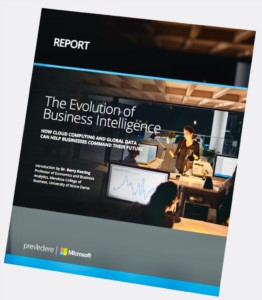
The problem with traditional forecasting techniques is they assume the future will repeat the past. Data teams need to go beyond traditional analysis techniques and find ways to account for major turning points that can change consumer behavior or supply chains. With the strategic use of relevant, quality data and business intelligence, companies are empowered to plan effectively.
More powerful forecasting models, like segmented multivariate analysis fueled by machine learning based software, outperform traditional processes in longer-term forecasts and can be used to identify leading indicators.
3. Embrace predictive analytics and machine learning
Predictive analytics and machine learning can greatly speed up the time it takes for teams to uncover big data and business intelligence insights and identify the unique forces that matter most for future performance.
However, the current data scientist skills shortage, particularly with those proficient in machine learning techniques, means many organizations may feel they lack the ability to successfully implement these technologies.
Pre-built predictive analytics and augmented analytics tools offer a promising solution for data teams. Vendors like Prevedere can help both data generalists and highly skilled data teams transform their organization’s data gathering and analysis processes.
Requiring less technical training to operate, these tools can free up valuable time and make business intelligence and demand planning efforts more effective.
As 2019 gets underway, contact us to learn more about how Prevedere can help your business create data-driven decisions. For additional ideas for data resolutions, download our Data Scientist’s Survival Guide.
***
Executives are hungry for meaningful, big data and business intelligence insights. Jobs with “analytics” in the title abound. It may seem as if there is no better time to be a data scientist, yet research shows that is not always the case. Data scientists report spending their valuable time heavily on “data” and not enough on “science.” Read the full report here.